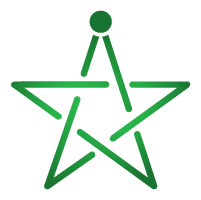
Lipostar 2.0
Data processing pipeline for untargeted lipidomics
Description
Lipostar is a software developed by Molecular Discovery for LC-MS/MS-based lipidomics (DDA and DIA), which supports a large number of steps including lipid identification, quantification, statistical analysis, and biopathways analysis. Lipostar finds application either in untargeted, and semi-targeted lipidomics, including stable isotope labelling experiments. Within a Lipostar session, different modes of lipidomics analysis can be combined to increase the knowledge and obtain a more comprehensive analysis of lipid profiles. Lipid identification includes 1) a spectral matching approach, with the DB Manager module allowing to generate databases of fragmented lipids by applying fragmentation rules provided in the software or by importing experimental MS/MS data; 2) a high-throughput bottom-up approach, based on class-specific fragments recognition; 3) a high-throughput identification of oxidized species. Lipostar also includes unique features, such as the gap-filler to reduce the missing values and the trend analysis for global lipid profiling.